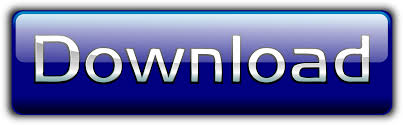

The best performances obtained by the SVM classifier using the essential features were 5-40% more than those using all of the retained features. In particular, the cortical thickness produced a classification with 98.84% accuracy, 97.5% sensitivity, and 100% specificity for the sMCI-cMCI comparison 92.37% accuracy, 84.78% sensitivity, and 97.22% specificity for the cMCI-NC comparison and 93.75% accuracy, 92.5% sensitivity, and 94.44% specificity for the sMCI-NC comparison.

The baseline cortical features combined with the longitudinal measurements for 2 years of follow-up data yielded prominent classification results. An optimized strategy of feature addition based on the weight of each feature was adopted for the SVM classifier in order to achieve the best classification performance.
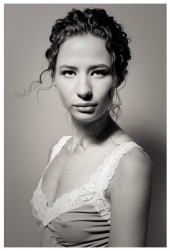
A sparse-constrained regression model based on the l2-1-norm was introduced to reduce the feature dimensionality and retrieve essential features for the discrimination of the three groups by using a support vector machine (SVM). In this study, 158 subjects (72 NC, 46 sMCI, and 40 cMCI) were selected from the Alzheimer's Disease Neuroimaging Initiative. Four cortical features, namely, gray matter volume, cortical thickness, surface area, and mean curvature, were explored for discriminative analysis among three groups including the stable MCI (sMCI), the converted MCI (cMCI), and the normal control (NC) groups. Cortical features and changes play a crucial role in revealing unique anatomical patterns of brain regions, and further differentiate MCI patients from normal states. Neuroimaging measurements derived from magnetic resonance imaging provide important information required for detecting changes related to the progression of mild cognitive impairment (MCI).
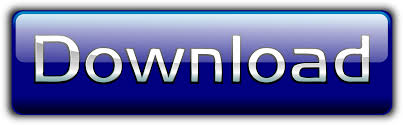